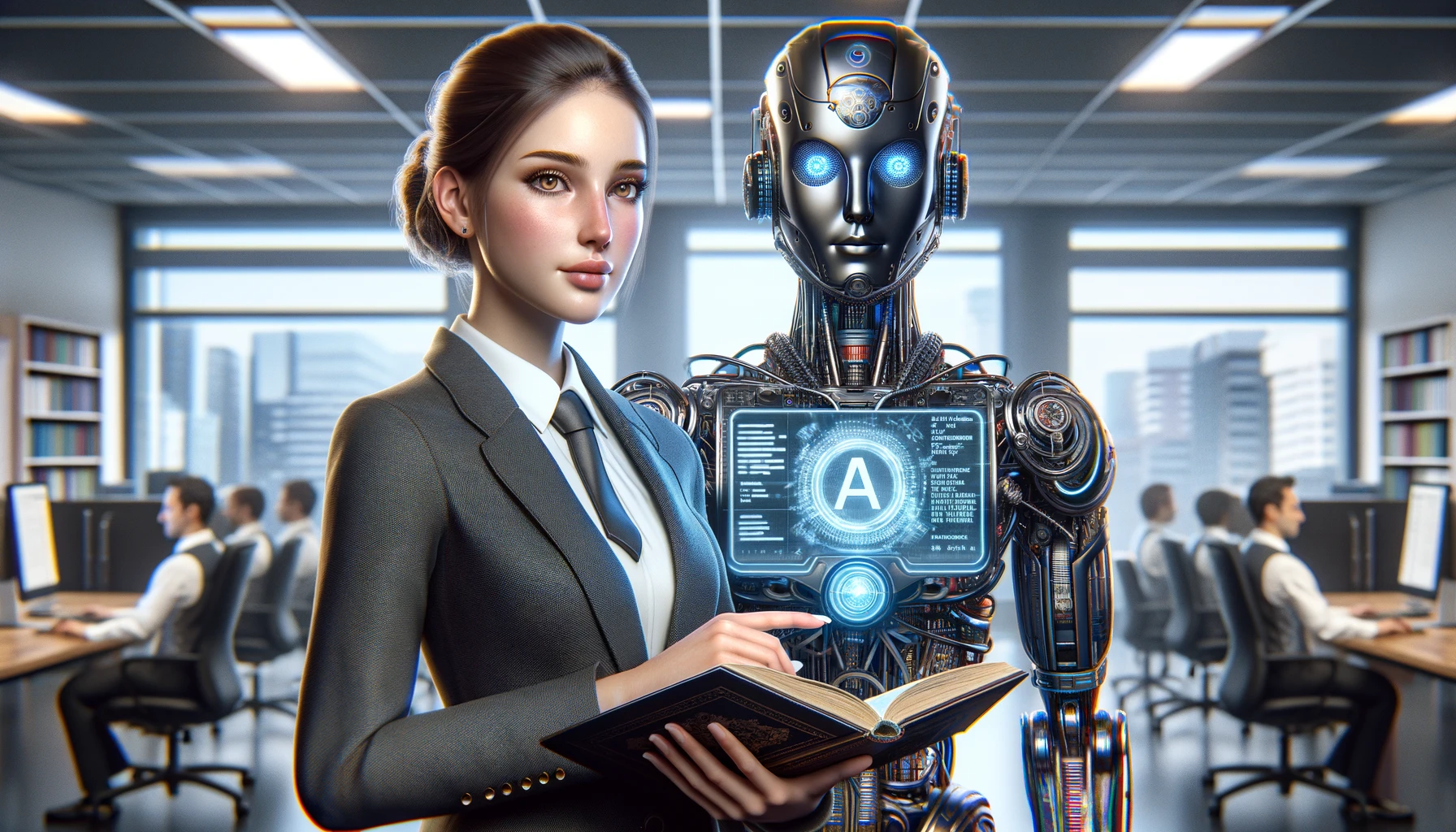
In the fast-evolving world of language services, the emergence of GenAI (Generative AI) is reshaping how we approach translation and localization. As industry thought leaders, it’s vital to understand and balance the potential of GenAI with the proven effectiveness of traditional methods.
What localization professionals are saying about GenAI
In the context of text generation, the appropriate term to use when talking about GenAI is LLMs (Large Language Models). In the following sections, we will use ChatGPT because that’s the LLM that most are familiar with. Several conversations are happening in the localization world about the opportunities and risks related to adopting ChatGPT and similar tools like Google Bard and DeepL. Some of the hottest topics are the following:
- There’s a debate about using ChatGPT instead of neural MT (Machine Translation) for Post-Editing. In the discussion, the comparison is not between traditional human translation and ChatGPT but between NMT (Neural Machine Translation) and ChatGPT.
- A few papers have been recently published testing the performance of ChatGPT as an MT Post-Editor against a human Post-Editor. According to these publications, GPT-4 reliably improved the raw MT output, but, of course, not to perfection. In this case, the comparison is between the human post-editor and ChatGPT.
- There’s a conversation among professionals in the localization field about using ChatGPT to generate target language content directly, such as using ChatGPT to accelerate transcreation.
- ChatGPT could also be used as a productivity tool by translators, project managers, and engineers. Microsoft Copilot and GitHub Copilot are great examples. Here, we’re comparing average workforce productivity with ChatGPT-enhanced productivity.
The GenAI risks for localization
The exciting discussions mentioned above have identified some general risk factors when using ChatGPT:
- Truthfulness. ChatGPT isn’t reliable for factual information. This means that the text generated by ChatGPT lacks a factual basis. Due to the way LLMs are designed and programmed, it’s not feasible to trace the origin of any statement or piece of information mentioned by ChatGPT.
- Making Sense. ChatGPT appears to have a high level of fluency that can sometimes conceal logical gaps. Although the text generated by ChatGPT might sound convincing, upon closer examination, we may observe some areas for improvement and even whole sentences that lack logical coherence.
- Not updated knowledge. ChatGPT knowledge is limited to events up to the date when it was trained before the release. Unlike Google, it doesn’t know about information released after that date. This limitation could lead to errors in accuracy and even legal issues if the output does not consider recent events.
- Limited knowledge. ChatGPT’s contextual understanding is limited to its training data. To some extent, a user can complement the original training data by uploading documents from the company’s knowledge base or mentioning specific facts directly in the prompt. ChatGPT recently introduced the option to create custom GPTs to address the issue. While custom GPTs can streamline the additional custom training phase, they also introduce severe vulnerabilities in handling and storing sensitive documents and information.
- Creativity. ChatGPT’s output may follow repetitive patterns when not properly directed, giving the perception of a lack of creativity. To gain complete control of this tool’s creativity, a user needs a deep understanding of the technology and a significant skill level in formulating the most effective prompts—something we can not reasonably expect from professional translators.
- Ethical concerns. Ethics constitute an essential risk when using ChatGPT. We know that biased datasets lead to biased outputs in race, gender, religion, and other areas. OpenAI, the company that makes ChatGPT, doesn’t make the list of documents, books, and websites used for the initial training of the LLM publicly available.
- Legal concerns. There are also critical legal issues. Like everybody else, companies like OpenAI are vulnerable to data breaches. Therefore, our interactions with ChatGPT could end up in front of the wrong eyes, revealing sensitive information to malicious attackers.
- Privacy Concerns. A significant issue originates from how OpenAi uses our private conversation to train ChatGPT further. Based on how LLMs work, some sensitive information we used to craft a prompt could resurface in the output generated for another user on a similar prompt. ChatGPT employs a single centralized model that is accessible and utilized by all users.
- Copyright Concerns. Finally, the debate is still going on about the copyright implications of how ChtaGPT uses the sources from its training. The major AI players are publicly committed to shielding the end users from potentially expensive legal sues. OpenAI created ‘Copyright Shield’ to cover legal fees for customers facing AI-related copyright claims. Similarly, Google has recently announced measures to shield users of its GenAI products from third-party copyright infringement claims.
When applying ChatGPT in any of the mentioned use cases, we still need to place a human in the driver’s seat who understands the tool’s weaknesses and risk factors. With a human in the driver’s seat, the conversation about ChatGPT and traditional localization is not so much “this or that” but “proceed with caution to apply ChatGPT where it makes sense.”
Conclusions
We believe that the future of localization lies in a synergistic approach that leverages GenAI and human skills. As we advance, we must protect our localization teams and budgets by making informed decisions about technology adoption. We aim to combine GenAI’s strengths with human sensitivity and skills to enrich our linguistic landscape.